Projects
Improving Flood Prediction Along the White Nile with Neural Networks
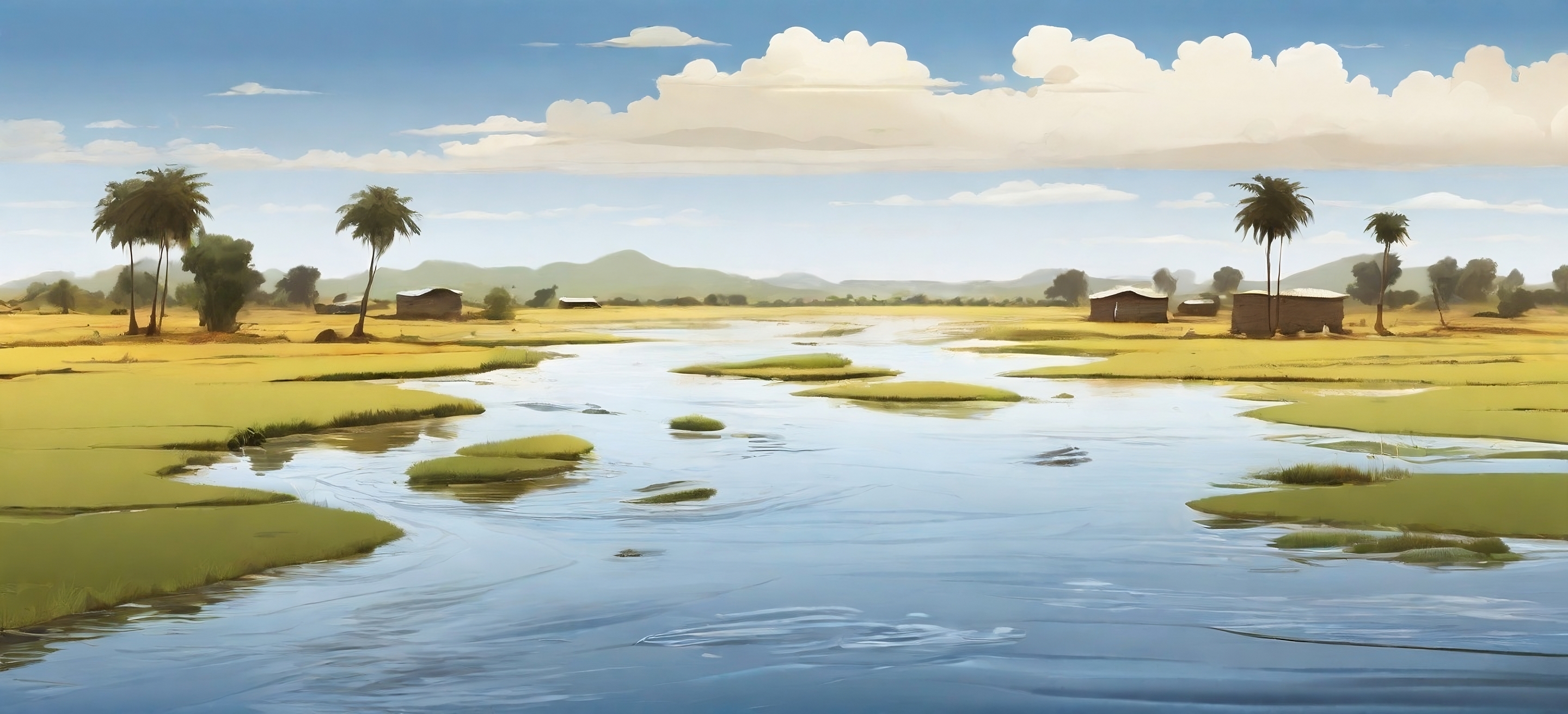
I built a two-stage neural network to automatically predict extreme out-of-sample inundation extent in the White Nile basin in South Sudan using satellite data from open sources.
Automating Typhoon Detection with StormSpyder
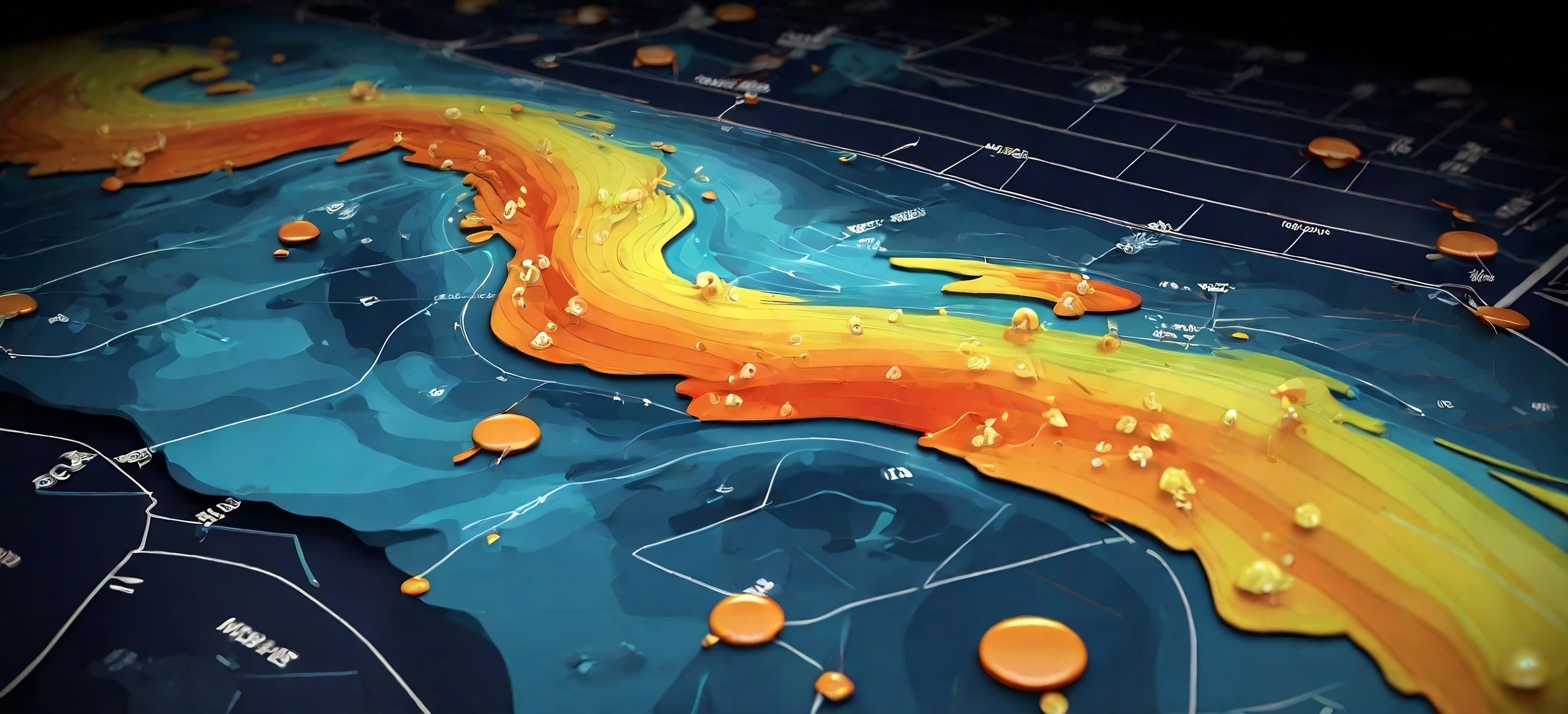
I developed a web-scraping tool to access the latest tropical storm forecasts from the European Centre for Medium-Range Weather Forecasts (ECMWF), detecting strike probabilities for tropical storms, tropical cyclones, and hurricanes up to nine days in advance. Results are emailed daily to key scientists at the FCDO.
Simulating Climate Policy Impacts by Building a Database of Emissions Research
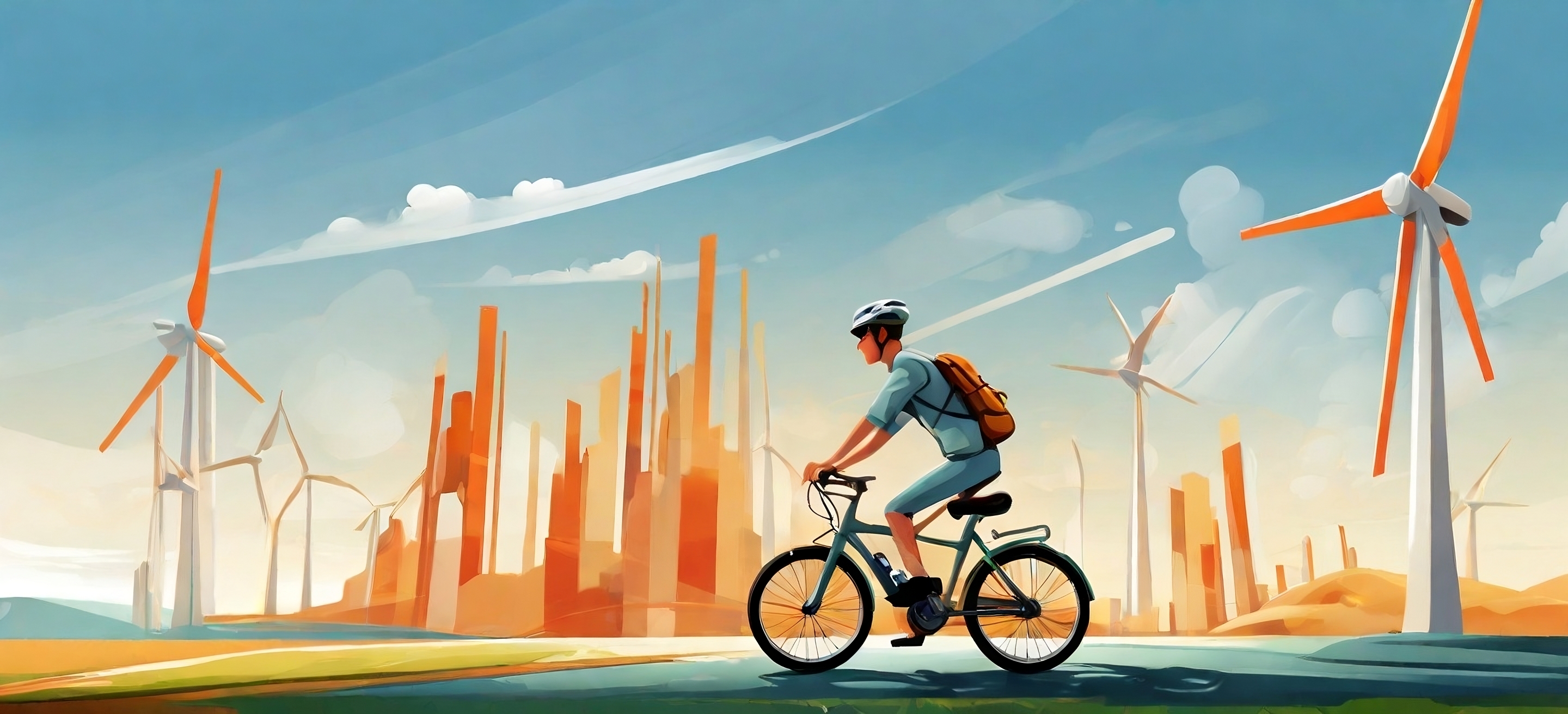
I led a team to collect data on the impact of various policy changes on global emissions and develop an R Shiny app to simulate which combinations of structural and behavioural changes need to be made to achieve Paris Agreement targets.
Monitoring Antimicrobial Resistance Using Large Language Models
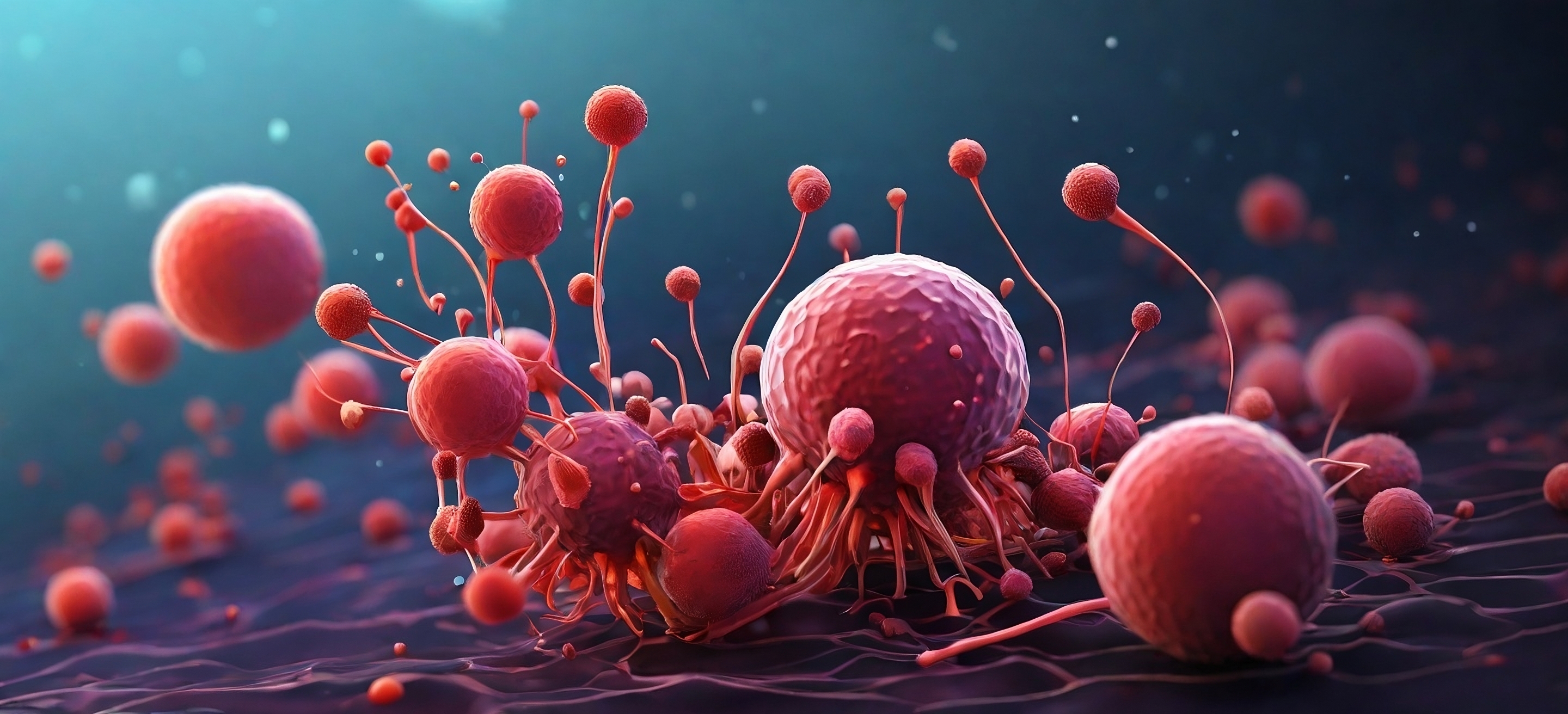
I led a team to use Selenium to scrape the internet for AMR-related stories around from regions of interest then apply public large language models to extract relevant information from the articles (type of threat, location, number of people affected, antibiotics administered etc.) for storage in a global AMR surveillance spreadsheet
Mapping Public Transit Systems in African Cities
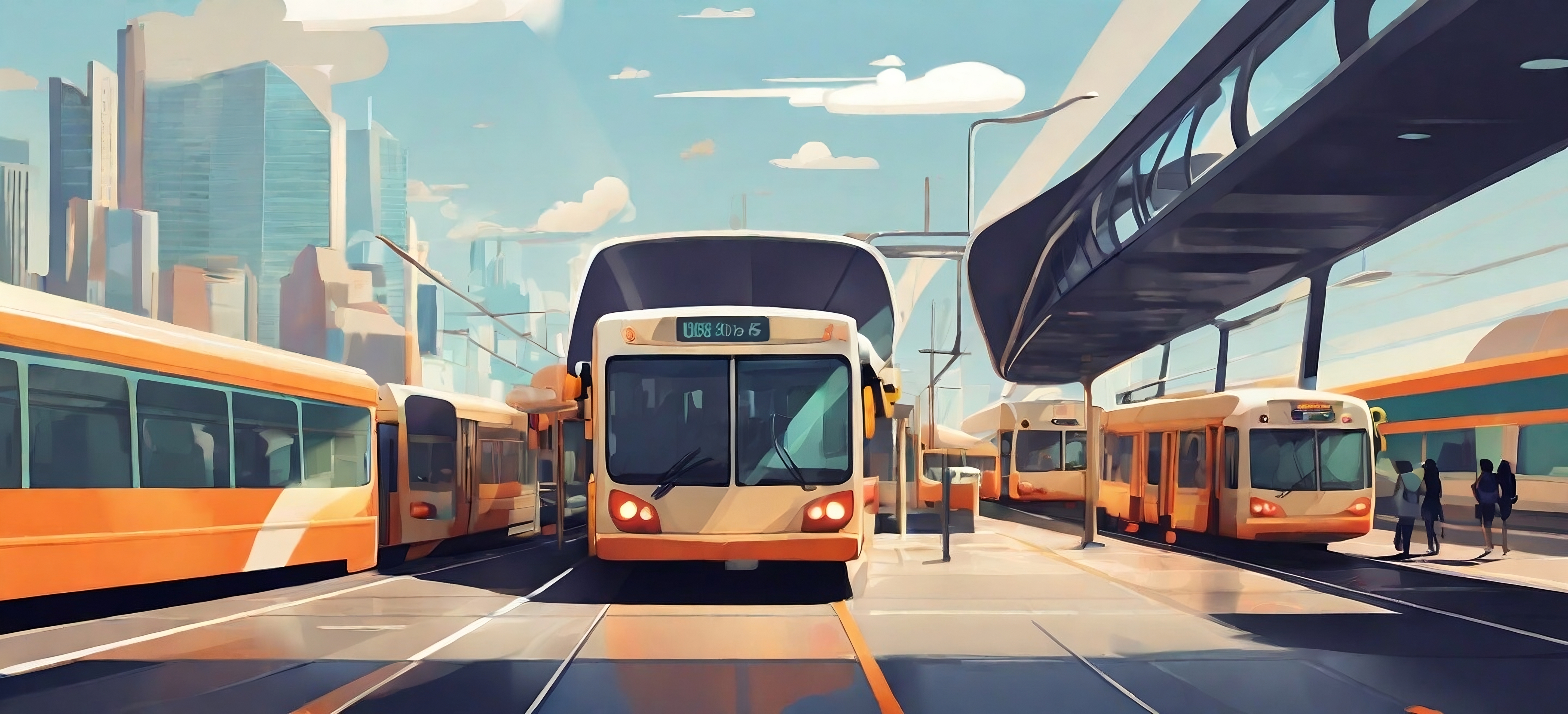
I led a research group to digitalise and analyse public transit routes in major Africa cities for DigitalTransport4Africa. The goal was to provide information to make public transit more accessible to residents and produce analytical insights for urban planners.
Simulating UBI Policy Impacts by Digitising Tax Policies
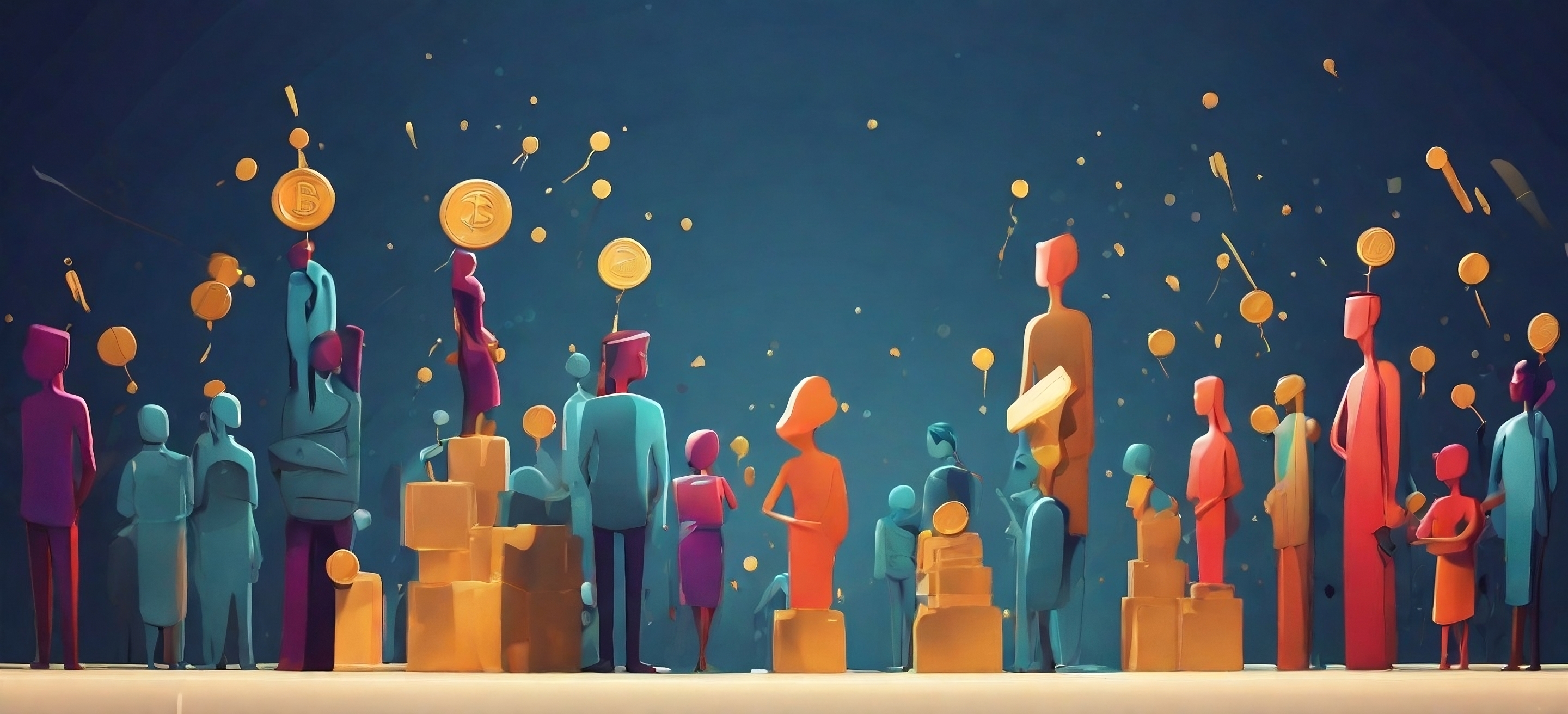
Universal basic income (UBI) has been proposed as a potential solution some of the biggest threats faced by modern workers: wage inequality, job insecurity – and the looming possibility of AI-induced job losses. I led a team to collect data on tax policies for G20 countries and develop an R Shiny app to simulate different taxation paradigms.
Predicting Typhoon Damage to Improve Disaster Response in the Philippines
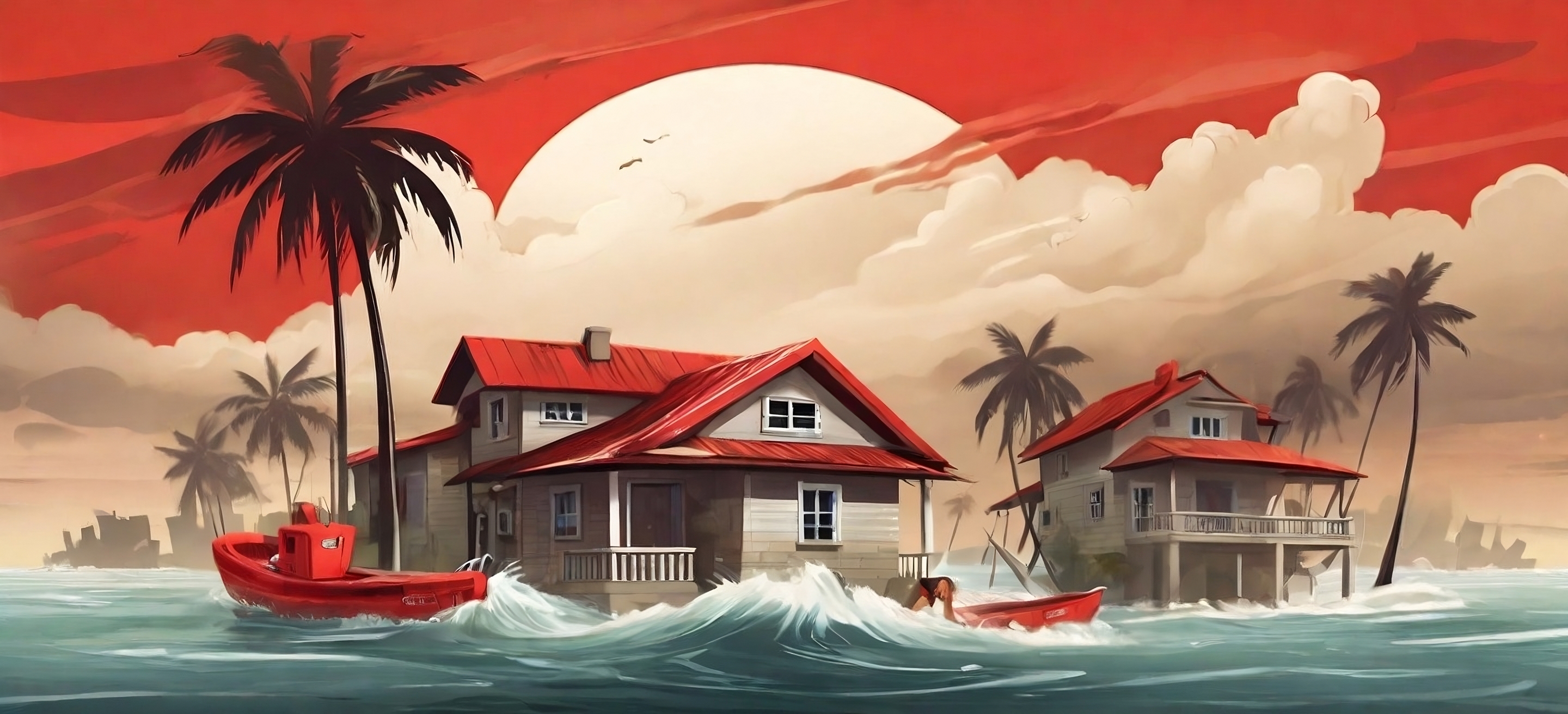
I am working with the Red Cross to improve their impact-based forecasting (IBF) model for typhoon damage in the Philippines. The goal is to make the model sensitive to errors in decision-making such differences in failing to protect an impacted municipality vs. spending money to unnecessarily protect an unaffected municipality.
Building a Neural Network to Address Canada’s Housing Crisis
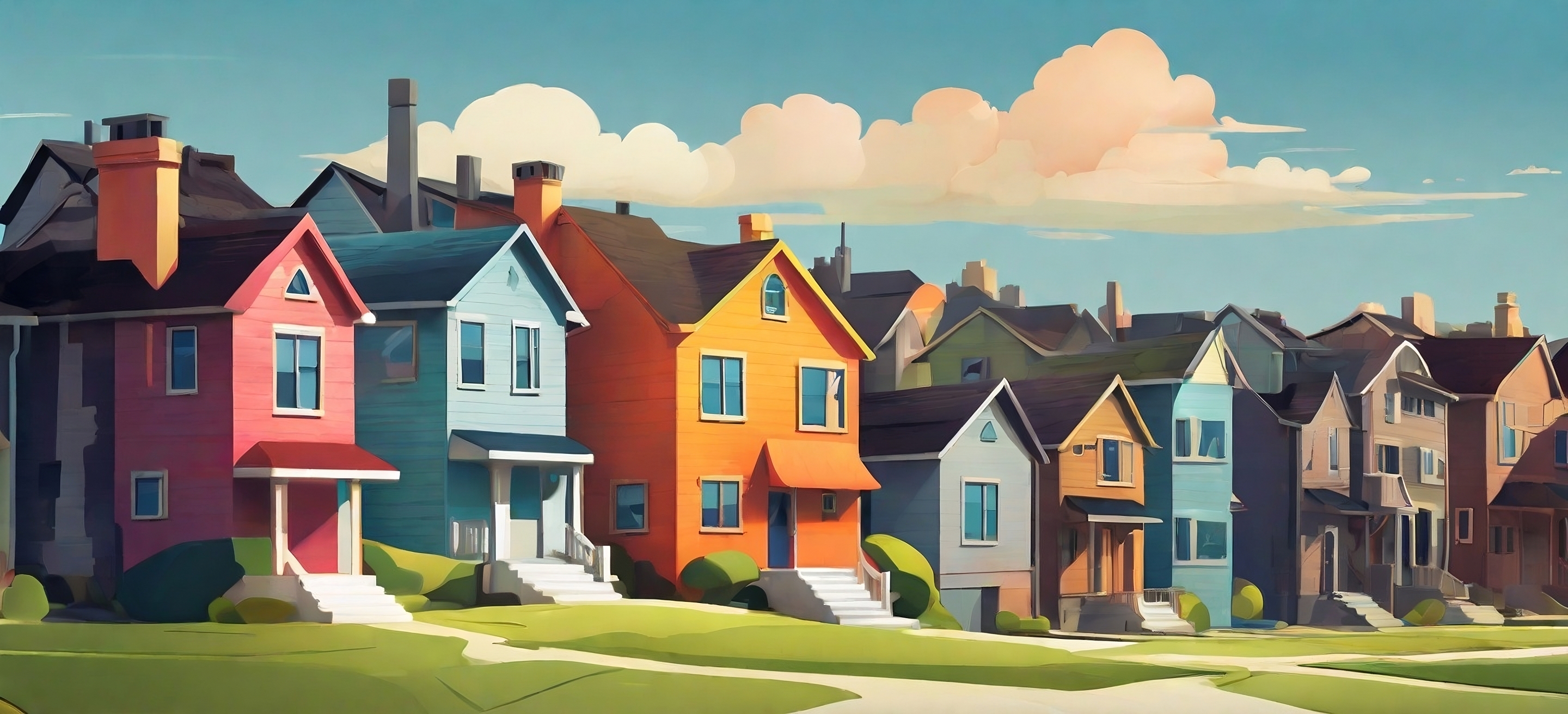
Increasingly unaffordable housing prices have become a major challenge for several developed countries, including Canada. I am leading a project to model the complex factors that contribute to these price changes using longitudinal data, enabling policy makers to understand how policy changes can impact housing prices.
Enhancing Crop Yield Predictions in Rural Bihar with XYieldBoost
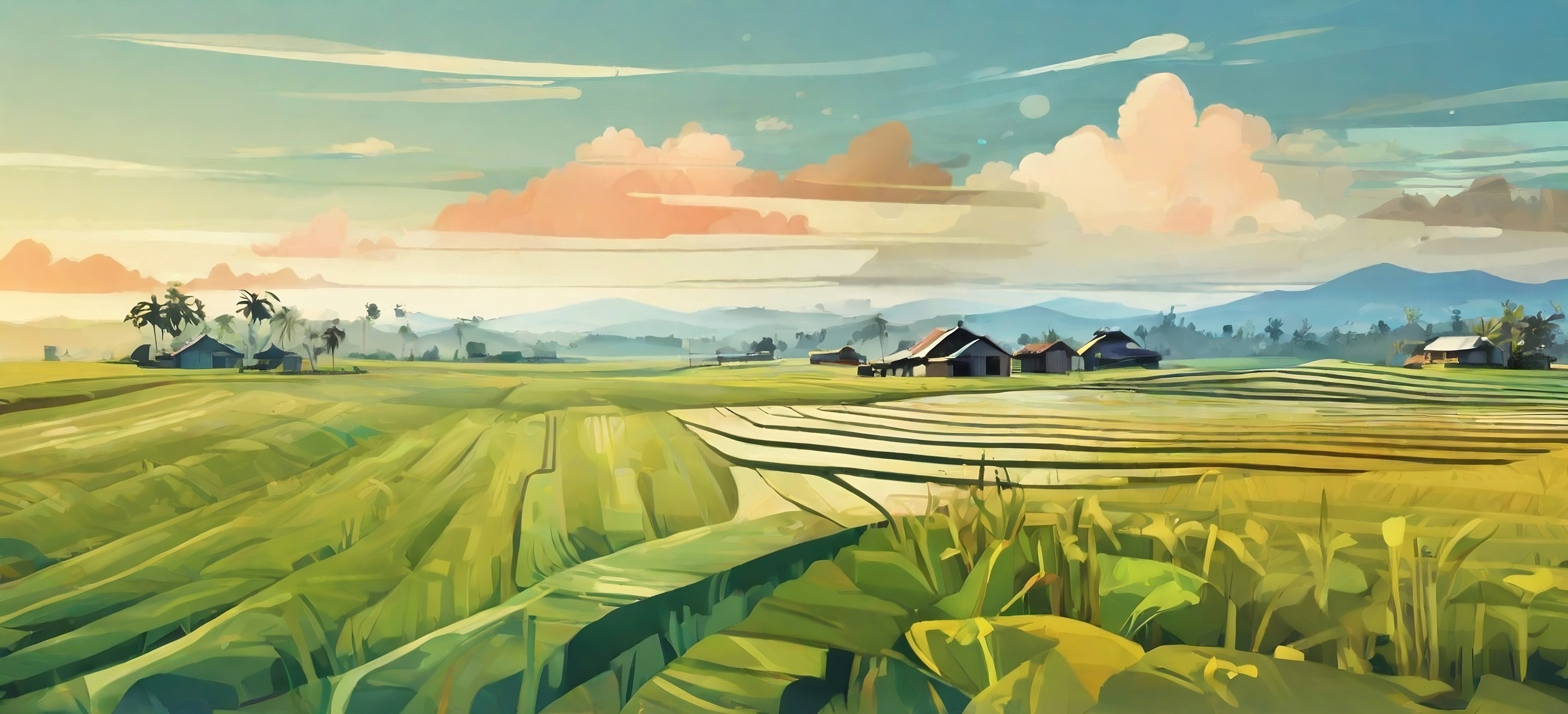
In collaboration with Digital Green, I led a team to build a machine learning solution that predicts the crop yield per acre of rice or wheat crops in Bihar. The goal is to empower smallholder farmers and break the cycle of poverty and malnutrition in northern India.
Generating Machine Learning-Based Streamflow Forecasts for the Western U.S.
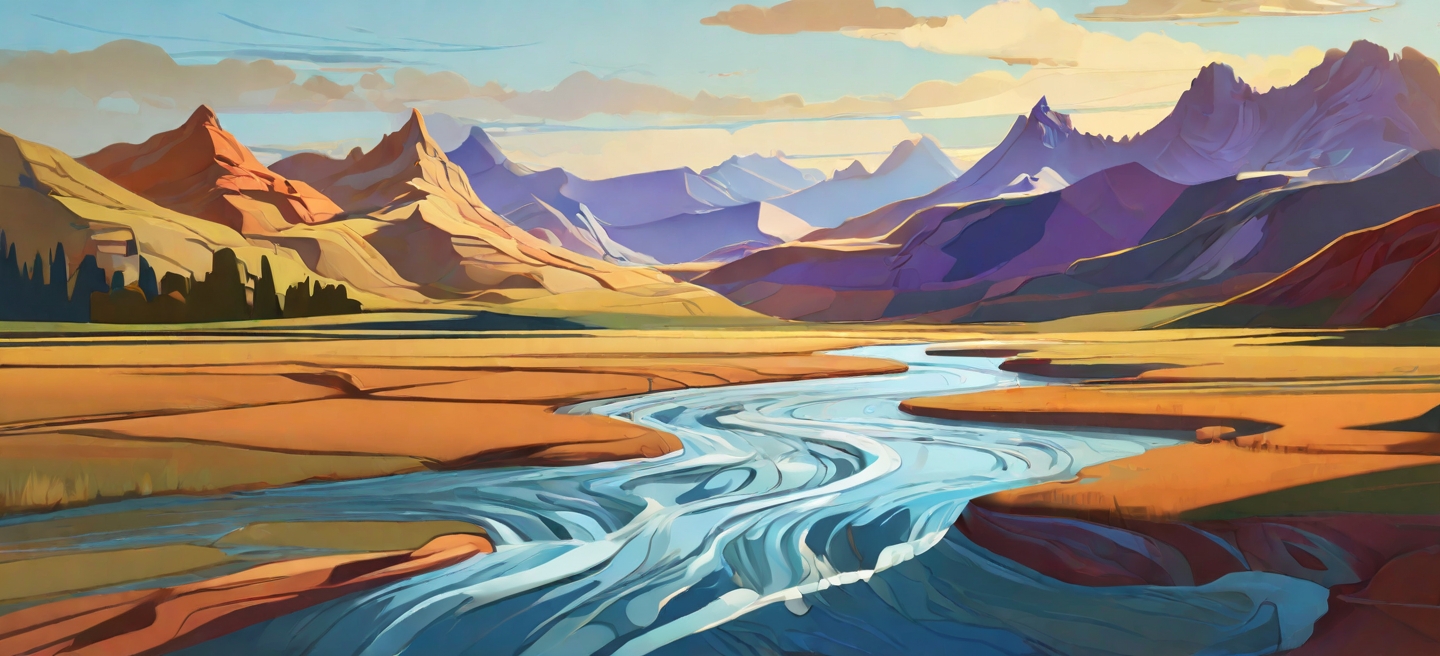
In collaboration with DrivenData and the United States Bureau of Reclamation (USBR), I led a team to develop a spatially-aware, explainable machine learning forecast seasonal streamflow in the Western United States.